After performing exploratory analyses such as PCA, UMAP and t-SNE is is helpful to visualize the results on a scatterplot. This can help visually assess the source of variation affecting the results of an experiment, classify cells and select samples for downstream analysis. Here we have a PCA scatterplot generated from the analysis of 12 samples from a scRNA sequencing study.
Numbered figure captions |
---|
SubtitleText | Example of a 3D PCA scatterplot . |
---|
AnchorName | Example of a 3D PCA scatterplot |
---|
|

|
The Configure > Style menu on the left can then be used to color the features in the scatterplot based on an attribute (Figure 2). In this case, Figure 3 shows the cells being colored based on their cell-type.
Numbered figure captions |
---|
SubtitleText | Customization menu. |
---|
AnchorName | Customization menu |
---|
|

|
Numbered figure captions |
---|
SubtitleText | PCA scatterplot colored by cell-type. |
---|
AnchorName | PCA scatterplot colored by cell-type. |
---|
|

|
Additionally, you can adjust the opacity of the points to better assess the density across the groups (Figure 4). It is also possible to split the plot based on the same attribute in the Configure > Grouping menu (Figure 5)
Numbered figure captions |
---|
SubtitleText | Adjusted opacity shows point density more accurately. |
---|
AnchorName | Adjusted opacity shows point density more accurately. |
---|
|
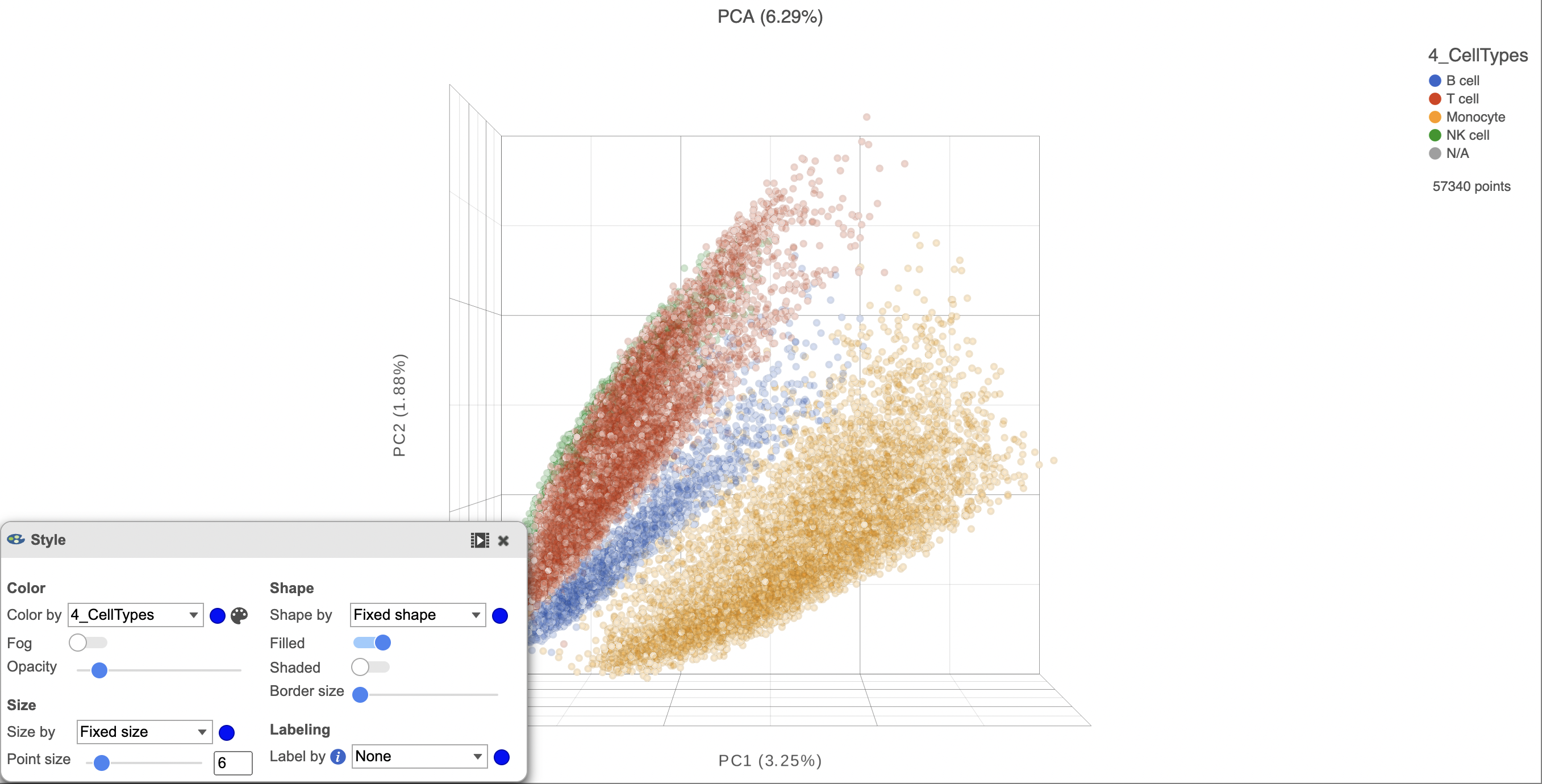
|
Numbered figure captions |
---|
SubtitleText | Splitting by an attribute can help better visualize their effect on the data. |
---|
AnchorName | Splitting by an attribute can help better visualize their effect on the data. |
---|
|
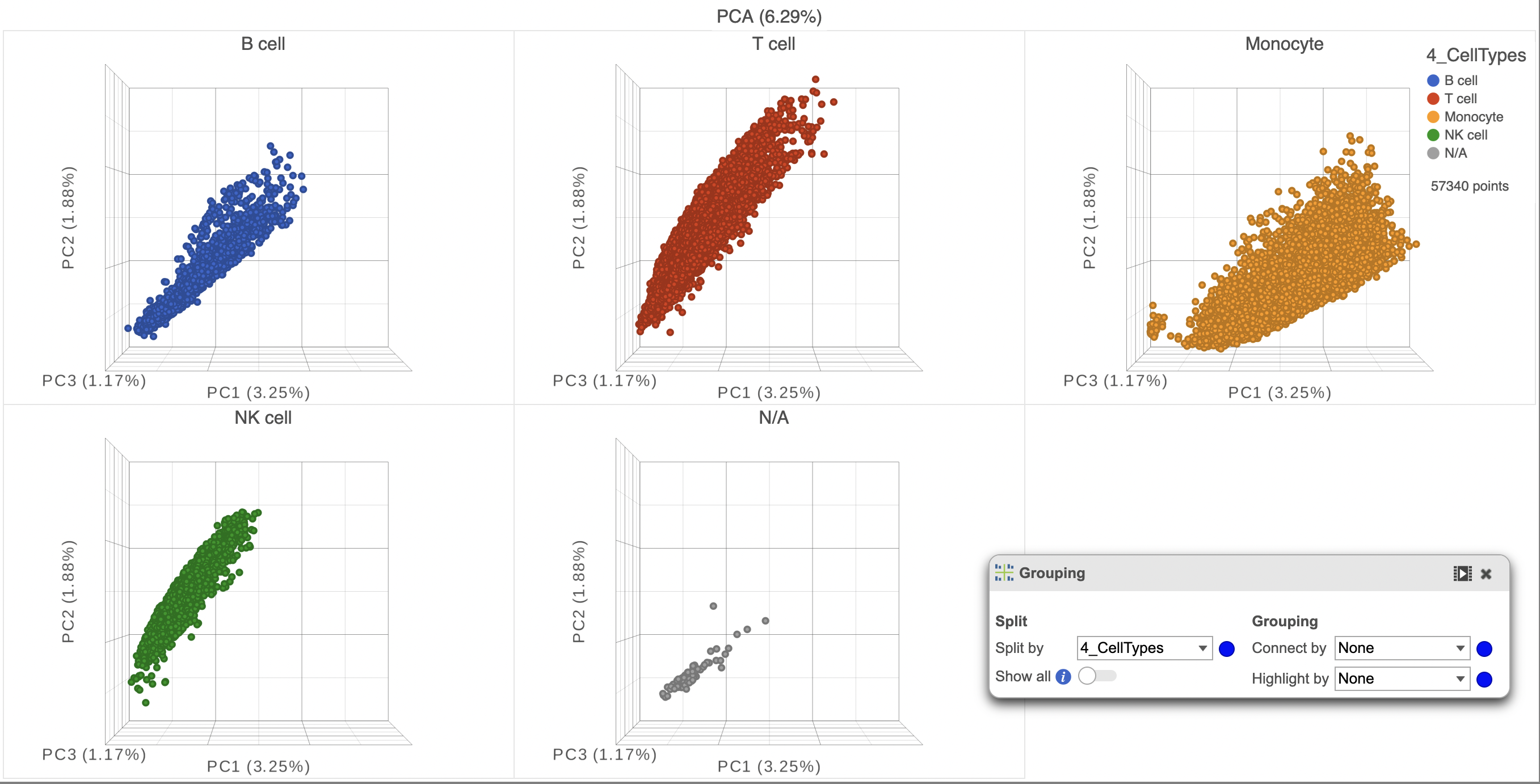
|
Click the Save image button
to save a PNG or SVG image to your computer.
Click the Send to notebook button
to send the image to a page in the Notebook.
ghjkl
ghjkl
ghjkl